Artificial intelligence is rapidly reshaping the AEC industry, driving innovations that promise to revolutionize the way projects are designed, managed, and executed. But behind every groundbreaking tool and strategic initiative is a network of forward-thinking professionals whose work with AI is paving the way for these changes.
In this series, we’ll be profiling leaders, innovators, and visionaries across the built world who are harnessing the power of AI to solve complex challenges, improve efficiency, and push the boundaries of what’s possible in the built environment. Our goal is to shine a light on their work, explore their perspectives, and highlight the transformative impact AI is having on the industry.
For the first profile in this series, we talked to Luis Lourenzi, lead data and AI product manager at Gerdau. Gerdau is the largest producer of long steel in the Americas, with mills spanning North and South American. Lourenzi shared his journey working with AI, applications for the technology in manufacturing, and emerging trends. Read below for more insights.
Edited for clarity.
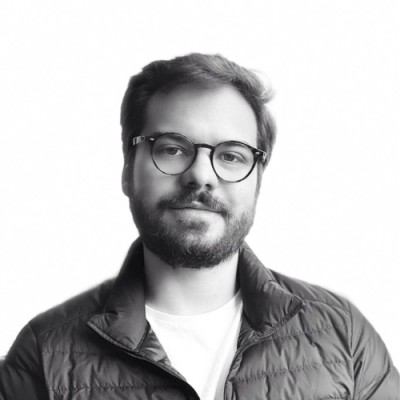
Could you please tell me about your current role and your journey within Gerdau? It looks like you've held several different positions.
I currently serve as the lead data and AI product manager at Gerdau, where I lead data product teams focused on creating solutions that align with our strategic business goals. My journey with the company began in 2017 as a product manager focused on digital solutions, building internal digital products. Over time, I transitioned into digital innovation and venture building. I led initiatives to diversify growth and implement digital ventures, aligning with the company’s strategy to expand beyond steel into other growth markets. My experience spans regions like the U.S. and Latin America, mainly focusing on digital transformation and innovation projects.
How did you transition to your current role?
I was working with innovation projects and trying to solve problems inside the steel world. I saw internal opportunities and market trends, with startups increasingly using AI and data solutions to solve industry problems. Instead of solely searching for external solutions, we pivoted towards building internal ones, especially when existing market solutions weren’t a perfect fit. This transition was smooth since I shifted from managing a portfolio of companies to building internal solutions for problems where we didn't necessarily have a solution out there.
Can you share any interesting projects you've worked on?
We've mostly built predictive maintenance models using AI and machine learning to analyze sensor data from equipment to predict failures before they occur. So instead of being reactive and doing maintenance on some of the equipment that we have across our mills, we are trying to analyze the patterns, create models to analyze that data, and provide insightful information, allowing us to have more proactive maintenance and avoid and plan for downtime.
Another initiative is the current use of generative AI. We were debating internally the use of generative AI, especially in regards to increasing employee productivity, so right now we're leveraging foundation models to improve employee workflows. One example is in HR. We’ve implemented an HR system that helps employees quickly get answers to everyday requests, freeing up HR time and improving employee happiness.
Have employees been comfortable using AI for their daily HR inquiries?
Initially, there was some concern about sharing information. But the questions people are asking may be personal but they are not sensitive, like what is the vacation policy. They know only the HR representatives can access these questions, so it simulates the approach of having an assistant there, but you are still free to reach out to HR. We’ve seen increased adoption from the pilots across different departments. We've been starting with the early adopters that are willing to use the technology and leverage it for their day-to-day usage.
How do you view innovation at Gerdau and within the industry more broadly?
Over the past five to 10 years, I've observed increased adoption of digital innovation with the large industrial heavy equipment manufacturing firms because they are focused on optimizing production and reducing costs. So I think in the last five to 10 years we have been seeing these use cases in areas like predictive maintenance, quality control, supply chain optimization, even energy and sustainability. So I think to continue to advance and have even greater efficiencies and innovations, I think that's the only way to move forward, integrating AI, but I think there's a lot of challenges to it.
Could you expand upon those challenges?
So I think one of the challenges we notice being a 100 year-old company is that we do have a lot of legacy systems and processes. I think one of the first steps that people have to have in mind when working with AI or data overall, is just ensuring that you have a very consistent data quality process that is being established. I think that process to embrace the culture of digital transformation and the use of AI begins with making sure you have quality data, that you're actually able to access that data first, before trying to do a very good AI use case.
Additionally, training and workforce development are essential parts of this process. In the past, the industry often tried to build impactful AI solutions without having the necessary data quality and governance in place. Now, we recognize that creating value begins with establishing these foundations first. Once the data is reliable and systems are connected, we can focus on developing valuable and impactful AI use cases that truly transform our operations.
Any more advice on implementing AI and data initiatives?
Take a startup mentality. It helps to gain buy-in from different stakeholders as well because you can show the value of one initiative incrementally. Rather than putting a team together for two years in large-scale projects without seeing results, we focus on starting with small manageable projects and demonstrating the value incrementally. It's a huge change to how we're approaching each scenario.
How do you envision the role of AI evolving in the industry over the next five to 10 years?
I think right now it's very hard to try to predict. Few could have foreseen the rapid rise of generative AI. I certainly didn't think about that, but it is here, and right now we're trying to adapt and trying to make sure we can work with that technology. What organizations can do best in the next couple of years is to look at the potential of AI, realize the value of AI as we have it today in a couple of use cases, and understand how we as an organization will be prepared to adapt and innovate. Having a structure in place, having a strategy in place, and making sure that the workflows and the organization are prepared to adapt to changes in the industr—I think that's the best thing that I can do for my team and also for the organization.
What are some current trends you are seeing with AI technology in the industry?
The trends that we're seeing are things I've mentioned like predictive analytics for maintenance quality control. I think the integration with AI and IoT devices where we can use, for example, gen AI connects to your IoT devices and then understand what's going on and what are some suggested actions from the gen AI perspective. The adoption of digital twins in a lot of these organizations to simulate and optimize production processes are uses that we're seeing growing. I think that will mature in the next five to 10 years for sure and that will pretty much enable all the industry to have a more advanced driven supply chain optimization that are crucial for the industry's efficiency and sustainability.
Discussion
Be the first to leave a comment.
You must be a member of the BuiltWorlds community to join the discussion.