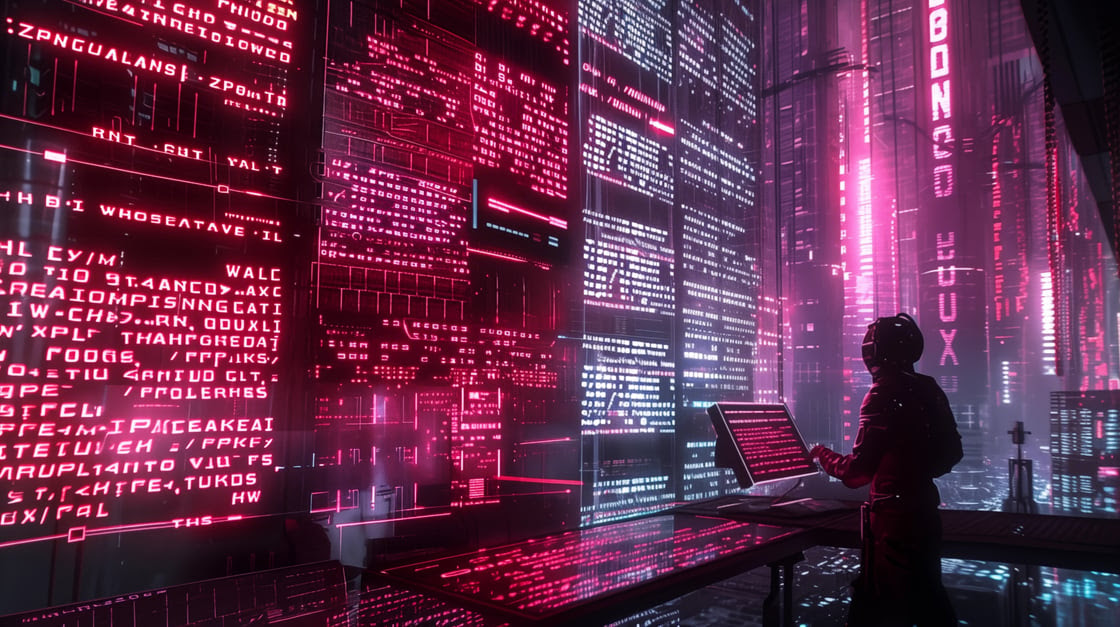
In the current venture capital landscape, financing AI is a bit like putting a kid through college; it requires a check that’s a lot bigger than it used to be, a few years of wondering how your money is being spent - maybe even a scare of having to finance another year - but your student graduates on time with a job at Goldman Sachs.
Amidst the AI boom of the past year-and-a-half, early-stage investment sizes for AI-enabled software solutions have been rivaling mid-stage funding rounds of asset-heavy and capital intensive startups like robotics, EVs or modular construction. These early-stage AI fundraises can range from $10-20M, even going as high as $150M.
- Exodigo, an AI company providing underground mapping for infrastructure and construction projects, closed $105M Series A funding co-led by Greenfield Partners and Zeev Ventures.
- Parspec, an AI-powered software platform that streamlines the selection and sales of construction products, closed $11.5M Seed funding led by Innovation Endeavors.
- Intenseye, AI-powered workplace safety solutions, closed $64M Series B led by Lightspeed Venture Partners.
Is there a departure in the capital requirements for AI software companies compared to traditional vertical SaaS startups? Like the parents of a college student, you may be wondering how this money is being spent.
Model Training and AI Infrastructure
The trend of massive, early-stage funding rounds in AI could simply be chalked up to AI hype, but that would be an unjust assessment.
Although it may be counterintuitive, AI-enabled startups are more asset heavy than their pure-play SaaS counterparts. An AI solution is only as good as its data. In order for an AI solution to operate, the model must be trained on prodigious amounts of data called large language models (LLM), which is essentially training a computer program to digest entire languages and generate human-like responses.
That’s a lot of data points to process and, consequently, requires a high-capacity of processing power. The infrastructure that enables LLM processing to occur are GPU computer chips that happen to be in short supply for the current level of demand.
As capital intensive and asset-heavy as this sounds for software companies, this is not the source of AI startups’ venture capital requirements. Parspec CEO Forest Flager elaborates, “while the training of models and digital infrastructure/hardware can also be expensive, we have found that most providers offer free or discounted services for early-stage startups through venture investors or other channels.”
Cloud computing providers like Amazon Web Services (AWS), Microsoft Azure and Google Cloud Platform (GCP) offer model training services that can be discounted or free for startups to use. Startups can also utilize pre-trained models developed by OpenAI, Microsoft and Google that offer a plug-and-play solution for startups adaptable to their business needs.
So if model training and AI infrastructure aren’t the major capital expenditures, then what is?
Talent Acquisition
Paradoxically, AI startups’ biggest use of capital is humans (so AI is not taking our jobs…yet).
“I believe the most challenging aspect of building an AI company today is convincing the best engineers to join your business given the myriad of options available to them. As a startup, you typically do not have the budget to compete with the cash being offered by tech giants like Google or Microsoft, so your vision must be compelling if you want to attract the best talent,” says Parspec CEO Forest Flager.
AI startups are competing with tech giants for a limited talent pool of software engineers that can code AI; startups need to get the right people in the door at the early stages to position themselves for long-term success. The talent acquisition component is revealing itself as the biggest hurdle for AI startups and thus requiring significant sources of capital to remain competitive.
Flager explains that a startup’s mission can be the differentiator in attracting top talent to an AI startup over Big Tech:
“At Parspec, we offer the opportunity to improve the affordability and sustainability of our built environment by empowering the construction industry to select and procure better building products and materials. Given that building construction constitutes approximately 4% of global GDP and 40% of greenhouse gas emissions, we have found this mission to be compelling for engineers who seek the opportunity to have a real impact on the world around them as well as financial gain. My co-founder and CTO Pratyush Havelia and I are tremendously proud of the team that we have assembled to achieve this mission.”
That prompts the next question: in the later-stages, will AI software funding resemble that of a vertical SaaS company once the talent is in place, the models have been trained and infrastructure acquired? There is not enough data yet to discern whether or not this is the case, but it will continue to be a research theme for BuiltWorlds Venture and Investments team.
As for those proverbial college parents, it may seem like they are paying for the whole freshman class to start, but in this case, it is the defining factor of success. Investors are willing to invest the capital for many of these early-stage ventures for the long-run value they propose.
Just be thankful your college student isn’t Sam Altman asking for $7 trillion.
Cameron Mabley is a Ventures and Investments analyst at BuiltWorlds.
Discussion
Be the first to leave a comment.
You must be a member of the BuiltWorlds community to join the discussion.